Note
Click here to download the full example code
Loading and Viewing the UCI Multiple Features Dataset¶
In this tutorial we demonstrate how to load and quickly visualize the Multiple Features Dataset [1] from the UCI repository, which is available in mvlearn. This dataset can be a good tool for analyzing the effectiveness of multiview algorithms. It contains 6 views of handwritten digit images, thus allowing for analysis of multiview algorithms in multiclass or unsupervised tasks.
[1] M. van Breukelen, R.P.W. Duin, D.M.J. Tax, and J.E. den Hartog, Handwritten digit recognition by combined classifiers, Kybernetika, vol. 34, no. 4, 1998, 381-386
# License: MIT
from mvlearn.datasets import load_UCImultifeature
from mvlearn.plotting import quick_visualize
Load the data and labels¶
Here We can load the entire dataset (all 10 digits). Then, visualize in 2D.
# Load entire dataset
full_data, full_labels = load_UCImultifeature()
print("Full Dataset\n")
print("Views = " + str(len(full_data)))
print("First view shape = " + str(full_data[0].shape))
print("Labels shape = " + str(full_labels.shape))
quick_visualize(full_data, labels=full_labels, title="10-class data")
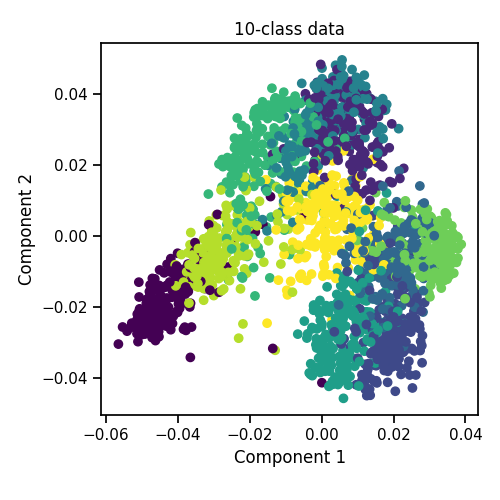
Out:
Full Dataset
Views = 6
First view shape = (2000, 76)
Labels shape = (2000,)
Load only 2 Classes of the Data¶
If we want only a binary classification setup, we can choose to only load 2 of the classes. Also, we can shuffle the data and set the seed for reproducibility. Then, we visualize in 2D.
partial_data, partial_labels = load_UCImultifeature(
select_labeled=[0, 1], shuffle=True, random_state=42)
print("\n\nPartial Dataset (only 0's and 1's)\n")
print("Views = " + str(len(partial_data)))
print("First view shape = " + str(partial_data[0].shape))
print("Labels shape = " + str(partial_labels.shape))
quick_visualize(partial_data, labels=partial_labels, title="2-class data")
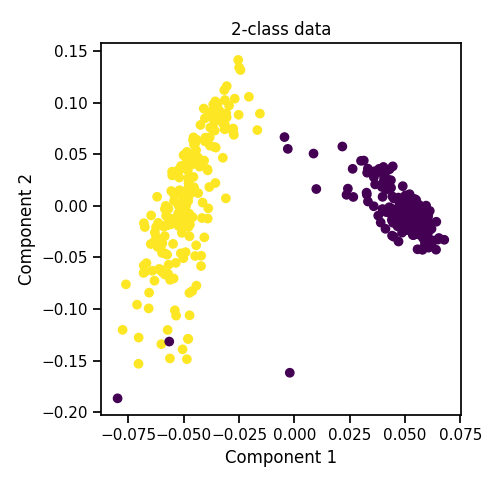
Out:
Partial Dataset (only 0's and 1's)
Views = 6
First view shape = (400, 76)
Labels shape = (400,)
Total running time of the script: ( 0 minutes 19.682 seconds)